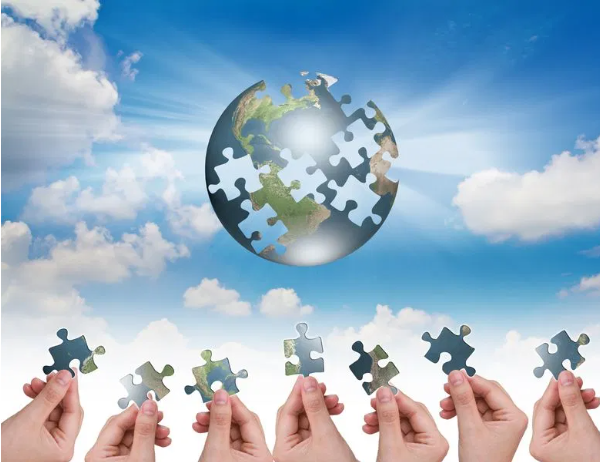
“We know there are known knowns; there are things we know we know. We also know there are known unknowns; that is to say we know there are some things we do not know. But there are also unknown unknowns—the ones we don’t know we don’t know” — Donald Rumsfeld
Ed Zuiderwijk, PhD
An Observation
There is something strange about climate models: they don’t converge. What I mean by that I will explain on the basis of historical determinations of what we now call the ‘Equilibrium Climate Sensitivity’ (ECS), also called the ‘Charney Sensitivity’ (ref 1), defined as the increase in temperature at the bottom of the Earth’s atmosphere when the CO2 content is doubled (after all feedbacks have worked themselves through). The early models by Plass (2), Manabe & co-workers (3) and Rowntree & Walker (4) in the 1950s, 60s and 70s gave ECS values from 2 degrees Centigrade to more than 4C. Over the past decades, these models have grown into a collection of more than 30 climate models brought together in the CMIP6 ensemble that forms the basis for the upcoming AR6 (‘6th Assessment Report’) of the IPCC. However the ECS values still cover the interval 1.8C to 5.6C, a factor of 3 difference in results. So after some 4 decades of development climate models have still not converged to a ‘standard climate model’ with an unambiguous ECS value; rather the opposite is the case.
What that means becomes clear when we consider what it would mean if, for instance, the astrophysicists found themselves in a similar situation with, for example, their stellar models. The analytical polytropic description of the early 20th century gave way years ago to complex numerical models that enabled the study of stellar evolution – caused by changing internal composition and the associated changes in energy generation and opacity – and which also in 1970, when I took my first steps in the subject, offered a reasonable explanation of, for example, the Hertzsprung-Russell diagram of star populations in star clusters (5). Although always subject to improvement, you can say that those models have converged to what could be called a canonical star model. The different computer codes for calculating stellar evolution, developed by groups in various countries, yield the same results for the same evolutionary phases, which also agree well with the observations. Such convergence is a hallmark of the progress of the insights on which the models are based, through advancement of understanding of the underlying physics and testing against reality, and is manifest in many of the sciences and techniques where they are used.
If the astrophysicists were in the same situation as the climate model makers, they would still be working with, for example, a series of solar models that predict a value of X for the surface temperature give or take a few thousand degrees. Or that, in an engineering application, a new aircraft design should have a wing area of Y, but it could also be 3Y. You don’t have to be a genius to understand that such models are not credible.
A Thesis
So much for my observation. Now what it means. I will here present my analysis in the form of a thesis and defend it with an appeal to elementary probability theory and a little story:
“The fact that the CMIP6 climate models show no signs of convergence means that, firstly, it is likely that none of those models represent reality well and, secondly, it is more likely than not that the true ECS value outside the interval 1.8-5.6 degrees.”
Suppose I have N models that all predict a different ECS value. Mother Nature is difficult, but she is not malicious: there is only one “true” value of ECS in the real world; if that were not the case, any attempt at a model would be pointless from the outset. Therefore, at best only one of those models can be correct. What is then the probability that none of those models are correct? We know immediately that N-1 models are not correct and that the remaining model may or may not be correct. So we can say that the a priori probability that any model is incorrect is [(N-1+0.5)/N] = 1–0.5/N. This gives a probability that none of the models is correct from (1-0.5/N)^N, about 0.6 for N>3. So that’s odds of 3 to 2 that all models are incorrect; this 0.6 is also the probability that the real ECS value falls outside the interval 1.8C-5.6C.
Now I already hear the objections. What, for example, does it mean that a model is ‘incorrect’? Algorithmic and coding errors aside, it means that the model may be incomplete, lacking elements that should be included, or, on the other hand, that it is over-complete with aspects that do not belong in it (an error that is often overlooked). Furthermore, these models have an intrinsic variation in their outcome and they often contain the same elements so those outcomes are correlated. And indeed the ECS results completely tile the interval 1.8C-5.6C and for every value of ECS between the given limits models can be found that can produce that result. In such a case one considers the effective number of independent models M represented by CMIP6. If M = 1 it means that all models are essentially the same and the 1.8C-5.6C is an indication of the intrinsic error. Such a model would be useless. More realistic is an M ~ 5 to 9 and then you come back to the foregoing reasoning.
A Little Story
Imagine a parallel universe (theorists aren’t averse to that these days) with an alternate Earth. There are two continents, each with a team of climatologists and their models. The ‘A’ team on the landmass Laputo has 16 models that predict an ECS interval 3.0C to 5.6C, a result, if correct, with major consequences for the stability of the atmosphere; the ‘B’ team at Oceania has 15 models that predict an ECS interval 1.8C to 3.2C. The two teams are unaware of each other’s existence, perhaps due to political circumstances, and are each convinced that their models set hard boundaries for the true value of the ECS.
That the models of both teams give such different results is because those of the A-team have ingredients that do not appear in those of the B-team and vice versa. In fact, the climatologists on both teams are not even aware of the possible existence of such missing aspects. After thorough analysis, both teams write a paper about their findings and send them, coincidently simultaneously, to a magazine published in Albion, a small island state renowned for its inhabitant’s strong sense of independence. The editor sees the connection between the two papers and decides to put the authors in contact with each other.
A culture shock follows. The lesser gods start a shouting match. Those in the A team call the members of the B team: ‘deniers’ who in their turn shout: ‘chickens’. But the more mature of both teams realize they’ve had a massive blind spot about things the other team knew but they themselves not. That those ‘unknowns’ had firmly bitten both teams in the behind. And the smartest realize that now the combined 31 models are a new A team to which the foregoing applies a fortiori: that there could arise a new B team somewhere with models that predict ECS values outside the 1.8C-5.6C range.
Forward Look
So it may well be, no, it is likely that once the underlying physics is properly understood, climate models will emerge that produce an ECS value considerably smaller than 1.8C. What could such a model look like? To find out we look at the main source of the variation between the CMIP6 models: the positive feedback on water vapour (AR4, refs 6,7). The idea goes back to Manabe & Wetherald (8) who reasoned as follows: a warming due to CO2 increase leads to an increase in the water vapour content. Water vapour is also a ‘greenhouse gas’, so there is extra warming. This mechanism is assumed to ‘amplify’ the primary effect of CO2 increase. Vary the strength of the coupling and add the influence of clouds and you have a whole series of models that all predict a different ECS.
There are three problems with the original idea. The first is conceptual: the proposed mechanism implies that the abundance of water vapour is determined by that of CO2 and that no other regulatory processes are involved. What then determined the humidity level before the CO2 content increased? The second problem is the absence of an observation: one would expect the same feedback on initial warming due to a random fluctuation of the amount of water vapour itself, and that has never been established. The third problem is in the implicit assumption that the increased water vapour concentration significantly increases the effective IR opacity of the atmosphere in the 15 micron band. That is not the case. The IR absorption by water vapour is practically saturated which makes the effective opacity, a harmonic mean, insensitive to such variation.
Hence, the correctness of the whole concept can be doubted, to say the the least. I consider therefore models in which the feedback on water vapour is negligible (and negative if you include clouds) as much more realistic. Water vapour concentration is determined by processes independent of CO2 abundance, for instance optimal heat dissipation and entropy production. Such models give ECS values between 0.5C and 0.7C. Not something to be really concerned about.
References
- J. Charney, ‘Carbon dioxide and climate: a scientific assessment’, Washington DC: National Academy of Sciences, 1979.
- G. N. Plass, ‘Infrared radiation in the atmosphere‘, American Journal of Physics, 24, 303-321 , 1956.
- S. Manabe and F. Möller, ‘On the radiative equilibrium and heat balance of the atmosphere‘ Monthly Weather Review, 89, 503-532, 1961.
- P. Rowntree and J. Walker, ‘Carbon Dioxide, Climate and Society‘: IIASA Proceedings 1978 (ed J. Williams), pages 181–191. Pergamon, Oxford, 1978.
- http://community.dur.ac.uk/ian.smail/gcCm/gcCm_intro.html
- V. Eyring et al, ‘The CMIP6 landscape’ Nature Climate Change, 9, 727, 2019.
- M. Zelinka, T. Myers, D. McCoy, et al. ‘Causes of higher climate sensitivity in cmip6 models‘, Geophysical Research Letters, 47, e2019GL085782, 2020. https://agupubs.onlinelibrary.wiley.com/doi/full/10.1029/2019GL085782.
- S. Manabe and R. Wetherald, ‘Thermal equilibrium of the atmosphere with a given distribution of relative humidity’, J. Atmos. Sci., 24, 241-259, 1967.