This post is inspired by an old post on the CERES cloud data by Willis Eschenbach that I’ve read and re-read a lot, “Estimating Cloud Feedback Using CERES Data.” The reason for my interest is I had trouble understanding it, but it looked fascinating because Willis was comparing CERES measured cloud data to IPCC modeled cloud feedback. I love obscure, back-alley comparisons of models and data. They tend to show model weakness. I learned this as a petrophysical modeler.
Willis wrote the post as a response to a paper by Paulo Ceppi and colleagues on cloud feedback in global climate models (Ceppi, Brient, Zelinka, & Hartmann, 2017). We’ll refer to the paper as Ceppi17. I took the time the last few days to understand Willis’ post and Ceppi’s paper and this is what I figured out, let me know what you think in the comments.
In Ceppi17, N = F + λΔT. N is the energy flux imbalance at the top of the atmosphere, F is a forcing, in W/m2 due to a sudden increase in greenhouse gases. The hypothetical situation used in the paper was a quadrupling of the CO2 instantly, relative to preindustrial conditions. Then they calculated a hypothetical F. “λ” is the cloud feedback and ΔT is the total global temperature change required to regain equilibrium, or an N of zero. Their feedback numbers cannot be duplicated with data due to the implausible scenario. Here are two more versions of the equation for reference.
ΔT = (N-F)/ λ or λ = (N-F)/ ΔT
What is N? N is a force imbalance between incoming (or downward) radiation and outgoing radiation at the top of atmosphere, which we will define as the CERES satellites. N is positive if the downward force is larger (warming) and negative if the outgoing radiation is larger (cooling). The Earth is at equilibrium if the feedback, N and F are zero. Positive feedback (λ) leads to warming and a larger imbalance (N). The higher the feedback, the greater the warming. If the feedback is negative, cooling or slower warming is the result.
“CRE” is the cloud-radiative effect, or the difference between the clear-sky and all sky radiative flux at the satellite (TOA). Clouds reflect incoming shortwave solar radiation (SW), so more SW travels up to the satellite in the presence of clouds, on average the increase is about -45 W/m2. This is a negative number because it means more radiation is leaving Earth, a cooling effect. Clouds also block some outgoing longwave infrared radiation (LW) emitted by Earth’s surface, on average about 27 W/m2, a positive number, since it is energy retained by Earth or less energy reaching the satellite, a warming influence. The difference is -18 W/m2, which means, overall, clouds cool the earth.
One would think that the more clouds, the more rapidly the earth would cool, but it isn’t that simple. Some clouds, especially low level clouds and cumulus clouds tend to reflect more energy during the day than they trap during the night. High level clouds, like cirrus, tend to allow solar SW through and trap a lot of upwelling LW, thus they have a warming effect. So cloud type matters.
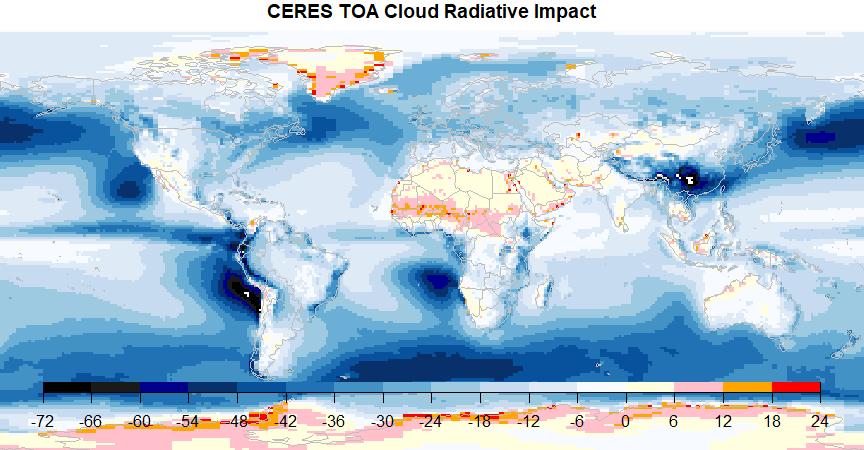
Figure 1. CRE in W/m2. Negative values (black, gray and blues) are cooling. Data from https://ceres.larc.nasa.gov/data/
Figure 1 is a map of the average TOA (top of the atmosphere) cloud-radiative imbalance (CRE) at the CERES satellites. The blues are a negative energy imbalance or a cooling CRE. The map is an average of CERES monthly data from 2001 through 2019. The CERES variable mapped is “toa_cre_net_mon” or the “Top of The Atmosphere Cloud Radiative Effects Net Flux.” The effect is negative (or cooling) everywhere, except over deserts and the polar land regions. These are areas where the clouds tend to trap surface and lower atmosphere infrared and simultaneously allow solar shortwave radiation through to the surface, this combination creates a positive CRE and strong warming.
The point where the cloud warming and cooling effects meet in the Figure 1 color scale is where the lightest blue meets the light yellow. Right at zero is where the incoming energy equals the outgoing energy, with respect to clouds. Thus, except for the Sahara, the Middle East, Western China, parts of Southeast Asia, Indonesia, Northern Australia, the Southwestern U.S., and Mexico, clouds cool the earth. The darker areas in Figure 1 have more persistent clouds.
Figure 2 has lighter colors for clouds and darker colors for clear skies. Thus the lighter streak, near the equator, in both the Pacific and the Atlantic, is white in Figure 2, opposite of Figure 1. This the Intertropical Convergence Zone (ITCZ) where the trade winds of the Northern Hemisphere and Southern Hemisphere converge. This is where evaporation of seawater is at a maximum. Water vapor is less dense than dry air so it is a zone of rapidly rising humid air and frequent rain and thunderstorms. It is almost always cloudy. The ITCZ follows the Sun’s zenith point and the cooling effect of the clouds in this zone is very high.
The maximum cloud cooling, or the most negative CRE values, are in the small white spots in the middle of the black spots off of southern Peru and in southeastern China, north of Vietnam. These CRE values are very negative (extremely cooling) and off-scale. Figure 1 correlates reasonably well with the cloud fraction in Figure 2, or the lighter colors in Figure 3, with the exception of the polar ice caps.
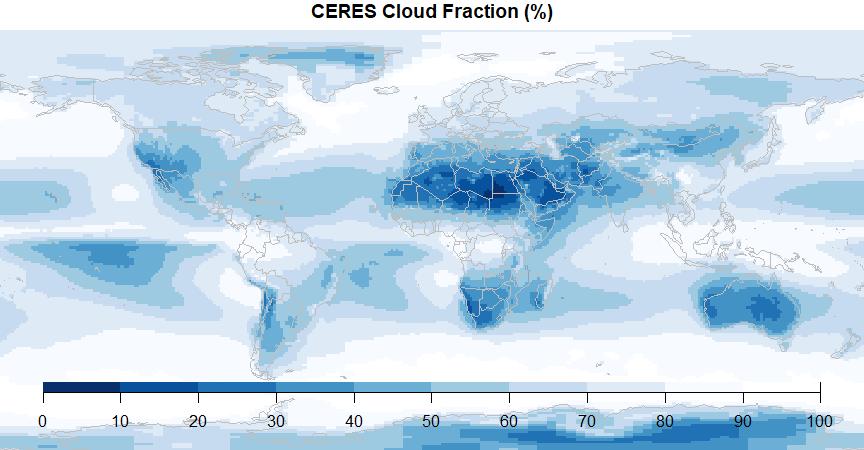
Figure 2. CERES cloud fraction in percent. Darker colors are less cloudy, lighter colors are more clouds.
Figure 3 is the NASA blue marble with ice and clouds shown in a Mercator projection. Notice the similarity with Figure 2, except at the poles.
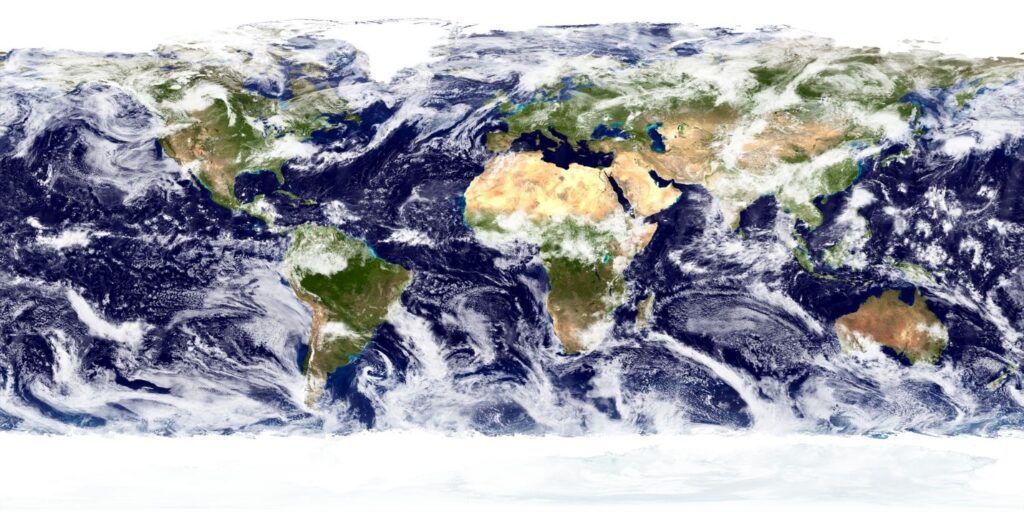
Figure 3. NASA blue marble with ice and clouds.
Figure 4 shows the same data, the CERES EBAF 4.1 variable “toa_cre_net_mon,” as yearly global averages. EBAF means energy balanced and filled. As Norman Loeb and colleagues (NASA Langley Research Center) explain, Earth’s energy imbalance is so tiny, between 0.5 and 1 W/m2, that it is only 0.15% of the total incoming and outgoing radiation. Thus, the number we are looking for is the difference between two large numbers and barely above the uncertainty in the satellite measurements.
The calibration uncertainty in the CERES SW measurement is 1% and 0.75% for the LW. Thus the outgoing LW is only known to ±2 W/m2. There are many other sources of error, and as Loeb, et al. explain, the net imbalance from the standard CERES data products is only ~4.3 W/m2, not much larger than the expected error. Due to the coarse resolution of the CERES instrument, there are a lot of missing grid cells in the one-by-one degree latitude and longitude grid used to make the maps in Figures 1 and 2. To get around these problems Loeb and colleagues use a complex algorithm to fill in missing values and adjust the SW and LW TOA fluxes, within their uncertainty ranges, to remove inconsistencies between the global net TOA energy flux and the heat storage in the earth-atmosphere system (Loeb, et al., 2018).
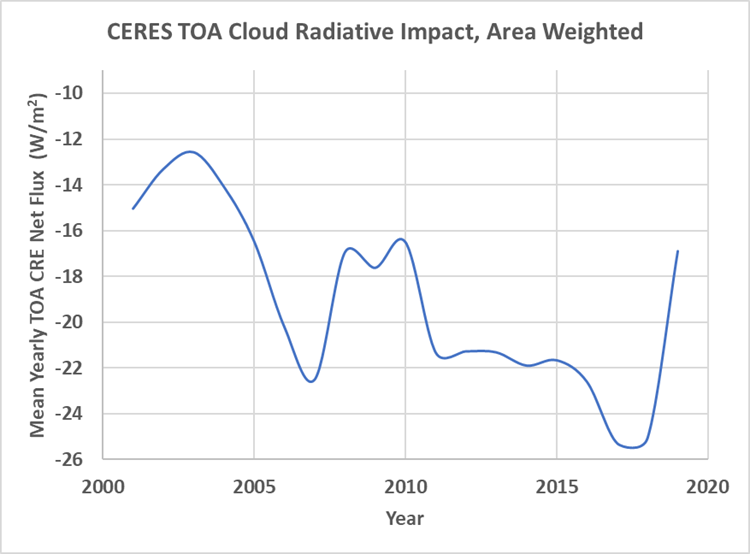
Figure 4. The CERES area-weighted average monthly TOA CRE (Cloud-Radiative Effect) from 2001 through 2019. Data from NASA.
The CRE, or cloud radiative imbalance value varies a lot from year to year, the average value over the 19 years is -19.1 W/m2, very close to the Ceppi, et al. value of -18 W/ m2 (Ceppi, Brient, Zelinka, & Hartmann, 2017). This suggests that total cloud cover is the main factor, it is shown below in Figure 5. As we would expect, as the cloud fraction goes down, the cooling effect decreases and the CRE becomes less negative. As the cloud fraction goes up the cooling effect increases.
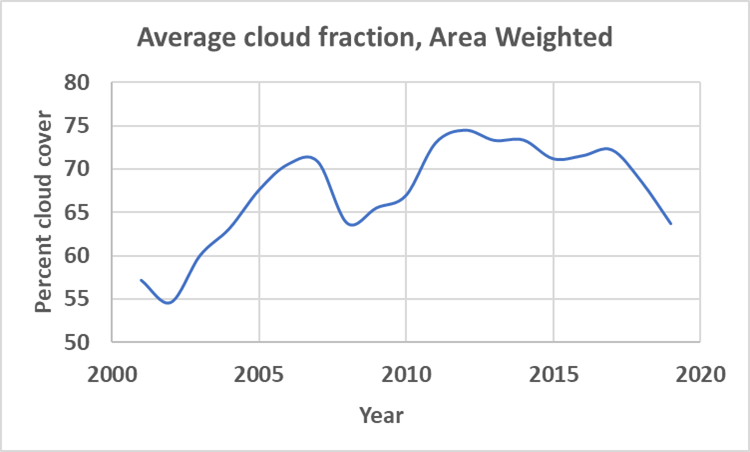
Figure 5. CERES Average monthly cloud fraction, variable cldarea_total_daynight_mon.
In Ceppi17 a more positive feedback parameter (λ) implies warming. Since they are working with models, they can compute λ by dividing the computed forcing required to counter the original forced energy imbalance, due to clouds, by the resulting temperature change (ΔT). Figure 6 shows Ceppi17’s feedback globally due to clouds.
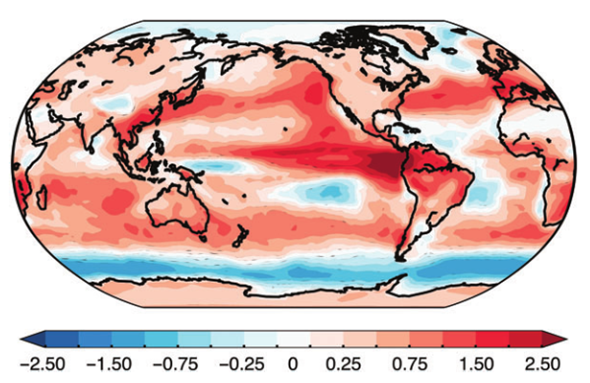
Figure 6. Ceppi17’s global cloud feedback parameter, the units are W/m2/K.
The units are W/m2/K, where K (Kelvin) is degrees C of warming or cooling due to clouds over the time it takes to reach equilibrium. Figure 6 is cloud feedback and not the same as CRE, but according to Ceppi17, cloud feeback tends to be positive and it suggests that clouds, over the long term, warm Earth and do not cool it. This made Willis question the whole paper. As he points out, Figure 6 is a plot of model output and Figure 1 is data. The data in Figure 1 is massaged and it is close to the edge in terms of uncertainty, but it is data.
Ceppi17 is lucky we cannot derive their feedback parameter from real data, because if we could, I suspect the map would look very different from Figure 6. For example, one of the places where clouds cool the surface the most is offshore of Peru, how does that turn into an area with a positive feedback? The other is southeastern China, OK, we get a little blue there, but nothing like what the actual data shows us. The very cloudy ITCZ is a very hot area in Figure 6, how do you do that?
I agree with Willis, this whole idea that clouds are a net positive (warming) feedback, makes no sense. The worst of it is that nearly every model uses a positive cloud feedback. Cloud feedback is the largest component of the model-computed ECS (the temperature sensitivity due to a doubling of the CO2 concentration) that the IPCC prefers. As we know, clouds cannot be modeled, and must be parameterized (the fancy modeling term for “assumed”). As Steve Koonin’s upcoming book, Unsettled, reports, scientists from the Max Planck Institute tuned their climate model by targeting an ECS of about 3°C by adjusting their cloud feedbacks. He adds “talk about cooking the books.” (Koonin, 2021, p. 93).
Ceppi17 reports that cloud feedback is, “by far, the largest source of intermodel spread in equilibrium climate sensitivity (ECS).” They also point out that cloud feedback is strongly correlated with model derived ECS and supply us with the data, it is plotted in Figure 7.
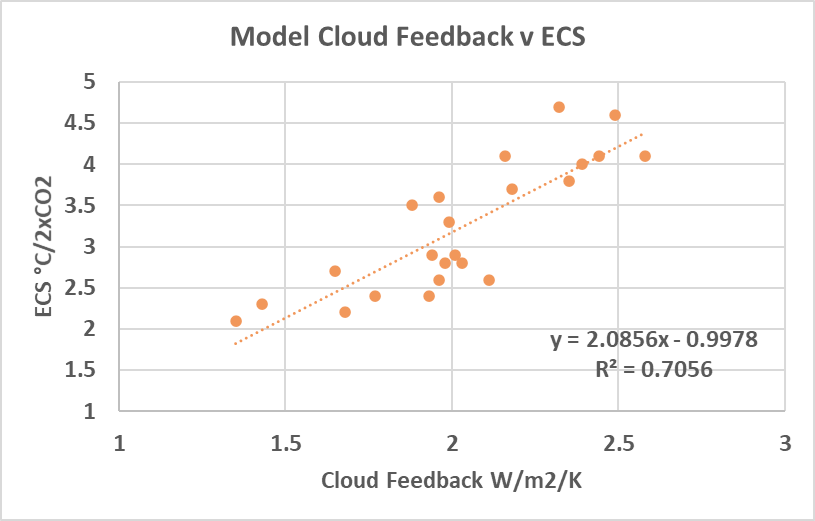
Figure 7. Modeled cloud feedback (λ) plotted versus model derived ECS. Data from (Ceppi, Brient, Zelinka, & Hartmann, 2017).
Oops! Clouds cannot be modeled, models assume their clouds have a warming effect, CERES says clouds have a net cooling effect, a large net cooling effect of -18 W/m2. The models say the entire human influence on climate since the beginning of the industrial era is 2.3 (1.1 to 3.3) W/m2 (IPCC, 2013, p. 661), which puts the cloud impact of -18 W/m2 into perspective. Notice that the variability in Figure 4 is larger than 2.3 W/m2. How much of the ECS from models is due to their assumption that clouds are net warming? How much is due to their assumption that ECS is 3 W/m2? So many questions.
Willis Eschenbach kindly reviewed this post for me and provided valuable input.
Works Cited
- Ceppi, P., Brient, F., Zelinka, M., & Hartmann, D. (2017, July). Cloud feedback mechanisms and their representation in global climate models. WIRES Climate Change, 8(4). Retrieved from https://onlinelibrary.wiley.com/doi/full/10.1002/wcc.465
- IPCC. (2013). In T. Stocker, D. Qin, G.-K. Plattner, M. Tignor, S. Allen, J. Boschung, . . . P. Midgley, Climate Change 2013: The Physical Science Basis. Contribution of Working Group I to the Fifth Assessment Report of the Intergovernmental Panel on Climate Change. Cambridge: Cambridge University Press. Retrieved from https://www.ipcc.ch/pdf/assessment-report/ar5/wg1/WG1AR5_SPM_FINAL.pdf
- Koonin, S. E. (2021). Unsettled: What Climate Science Tells us, What it doesn’t, and why it matters. Dallas, Texas, USA: BenBella. Retrieved from https://www.amazon.com/dp/B08JQKQGD5/ref=dp-kindle-redirect?_encoding=UTF8&btkr=1
- Loeb, N. G., Doelling, D., Wang, H., Su, W., Nguyen, C., Corbett, J., & Liang, L. (2018). Clouds and the Earth’s Radiant Energy System (CERES) Energy Balanced and Filled (EBAF) Top-of-Atmosphere (TOA) Edition-4.0 Data Product. Journal of Climate, 31(2). Retrieved from https://journals.ametsoc.org/view/journals/clim/31/2/jcli-d-17-0208.1.xml
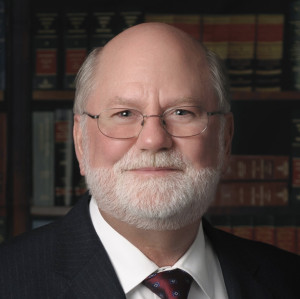
Andy May, now retired, was a petrophysicist for 42 years. He has worked on oil, gas and CO2 fields in the USA, Argentina, Brazil, Indonesia, Thailand, China, UK North Sea, Canada, Mexico, Venezuela and Russia. He specializes in fractured reservoirs, wireline and core image interpretation and capillary pressure analysis, besides conventional log analysis. He is proficient in Terrastation, Geolog and Powerlog software. His full resume can be found on linkedin or here: AndyMay