In my last post, on Scafetta’s new millennial temperature reconstruction, I included the following sentence that caused a lot of controversy and discussion in the comments:
“The model shown uses a computed anthropogenic input based on the CMIP5 models, but while they use an assumed climate sensitivity to CO2 (ECS) of ~3°C, Scafetta uses 1.5°C/2xCO2 to accommodate his estimate of natural forcings.”
I thought in the context of the post, the meaning was clear. But, Nick Stokes, and others, thought I meant that ECS was an input parameter to the CMIP5 climate models. This is not true, ECS is computed from the model output. If you pull the above quote out of the post and view it in isolation, it can be interpreted that way, so I changed it to the following which is unambiguous on the point.
“The model shown uses a computed anthropogenic input based on the CMIP5 models, but while they use an assumed climate sensitivity to CO2 (ECS computed from the CMIP5 ensemble model mean) of ~3°C, Scafetta uses 1.5°C/2xCO2 to accommodate his estimate of natural forcings.”
Then we received criticism about the computation of the ensemble model mean ECS, some said the IPCC did not compute an ensemble mean of ECS. This is nonsense, they compute it in AR5 (IPCC, 2013, p. 818). A portion of the table is shown below as Figure 1.
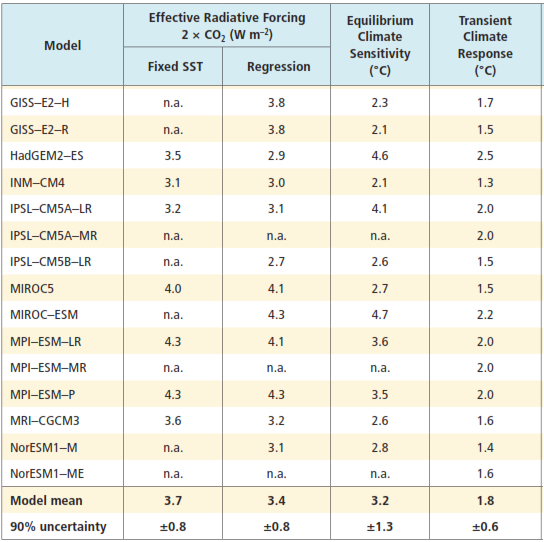
Figure 1. A portion of IPCC AR5 WG1 Table 9.5, page 818. The average ECS of the CMIP5 models is shown at the bottom as 3.2 degrees.
As you can see in Figure 2, most of the models greatly overestimate warming in the mid to upper tropical troposphere. A pressure of 300 hPa occurs at about 30,000 feet or 10 km altitude and 200 hPa is at about 38,000 feet or 12 km altitude. The top of the troposphere is the tropopause, and in the tropics, it is usually between 150 hPa or 14 km and 70 hPa or 18 km.
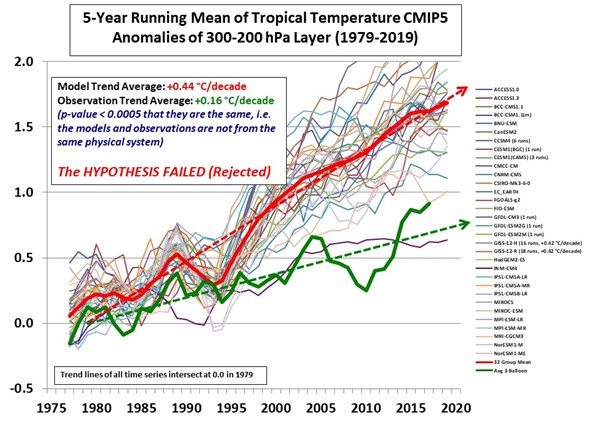
Figure 2. CMIP5 models versus weather balloon observations in green in the mid- to upper troposphere. The details of why the models fail statistically can be seen in a 2018 paper by McKitrick and Christy here.
The purple line in Figure 2 that tracks the weather balloon observations (heavy green line), is the Russian INM-CM4 model. As we can see, INM-CM4 is the only model that matches the weather balloon observations reasonably well, yet it is an outlier among the other CMIP5 models. Because it is an outlier, it is often ignored. In Figure 1 we can see that if ECS is computed from the INM-CM4 output, we get 2.1°C/2xCO2 (degrees warming due to doubling the CO2 concentration). Yet, while an ECS of 2.1 is clearly matching observations since 1979, the model average is 3.2. It is significant, literally, that INM-CM4 is one of the few models that passes the statistical test used in McKitrick and Christy, 2018 (see their Table 2). This is why I used the word “assumed.” The evidence clearly says 2.1, so 3.2 must be assumed. ECS is not an input to the models, but tuning the models changes ECS and the modelers closely watch the value when tuning their models (Wyser, Noije, Yang, Hardenberg, & Declan O’Donnell, 2020).
McKitrick and Christy chose the tropical middle to upper troposphere for their comparison very carefully and deliberately (McKitrick & Christy, 2018). This part of the atmosphere is sometimes called the tropospheric “hot spot” (See Figure 3). Basic physics and the IPCC climate models suggest that, if greenhouse gases (GHGs) are causing the atmosphere to warm, this part of the atmosphere should warm faster than the surface. Dr. William Happer has estimated that the rate of lower to middle tropospheric warming should be about 1.2 times the warming at the surface.

The reason is simple. If GHGs are causing the surface to warm, evaporation will increase on the ocean surface. Evaporation and convection are the main mechanism for cooling the surface because the lower atmosphere is nearly opaque to most infrared radiation. The evaporated water vapor carries a lot of latent heat with it as it rises in the atmosphere. The water vapor must rise because it has a lower density than dry air.
As it rises through the lower atmosphere, the air cools and eventually it reaches a height where it condenses to liquid water or ice (the local cloud height). This causes a tremendous release of infrared radiation, some of this radiation warms the surrounding air and some goes to outer space. It is this release of “heat” that is supposed to warm the middle troposphere. Does the “hot spot” exist? Theory says it should, if GHGs are warming the surface significantly. But proof has been elusive. In Figure 4 we plot the surface temperature from the ERA5 weather reanalysis versus the reanalysis temperature at 300 mb (also 300 hPa or about 10 km). The curves below are for most of the globe, the data is from the KNMI climate explorer. I tend to trust reanalysis data, after all it is created after the fact and compared to thousands of observations around the globe. This plot is one example, you can make others easily on the site.
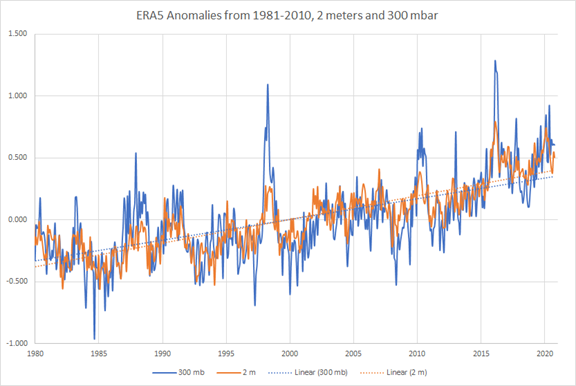
Figure 4. ERA5 weather reanalysis temperatures from the surface (2 meters) in orange and at 300 mbar (10 km). We expect a faster rate of warming at 300 mbar than at the surface, but, instead, the rates are almost the same, with the surface rate slightly higher. The El Niños have a higher rate at 300 mbar, which makes sense.
Surface ocean warming should cause a “hot spot.” We see this in every El Niño in Figure 3. Surface warming due to GHGs should do the same thing, but this is not seen in Figure 4. As I stated above, the models have been tuned to produce an assumed ECS.
Discussion
As a former petrophysical computer modeler, I’m surprised that CMIP5 and the IPCC average results from different models. This is very odd. Standard practice is to examine the results from several models and compare them to observations, this is what John Christy has done in Figure 2. Other comparisons are possible, but his is carefully done to highlight the differences. The spread in model results is huge, some go off scale in 2010. This is not a dataset one should average.
When I was a computer modeler, we would choose one model that appeared to be the best and average multiple runs from just that model. We never averaged runs from different models, it makes no sense. They are incompatible. I still think choosing one model is the “best practice.” I’ve not seen an explanation for why the CMIP5 produces an “ensemble mean.” It seems to be an admission that they have no idea what is going on, if they did they would choose the best model. I suspect it is a political solution for a scientific problem.
Also, the results (see Figures 1 and 2) suggest that the models are out of phase with one another. Figure 2 is a pile of spaghetti. Since it is obvious that natural variability is cyclical (see (Wyatt & Curry, 2014), (Scafetta, 2021), (Scafetta, 2013), and Javier’s posts here and here), this odd practice of averaging out-of-phase model results completely wipes out natural variability and makes it appear nature plays no role in climate. Once you do that, you have no valid way of computing the human impact. They have designed a method that guarantees the computation of a large ECS. Sad.
Works Cited
IPCC. (2013). In T. Stocker, D. Qin, G.-K. Plattner, M. Tignor, S. Allen, J. Boschung, . . . P. Midgley, Climate Change 2013: The Physical Science Basis. Contribution of Working Group I to the Fifth Assessment Report of the Intergovernmental Panel on Climate Change. Cambridge: Cambridge University Press. Retrieved from https://www.ipcc.ch/pdf/assessment-report/ar5/wg1/WG1AR5_SPM_FINAL.pdf
McKitrick, R. & Christy, J., 2018, A Test of the Tropical 200- to 300-hPa Warming Rate in Climate Models, Earth and Space Science, 5:9, p. 529-536
Scafetta, N. (2021, January 17). Climate Dynamics. Retrieved from https://doi.org/10.1007/s00382-021-05626-x
Scafetta, N. 2013, “Discussion on climate oscillations: CMIP5 general circulation models versus a semi-empirical harmonic model based on astronomical cycles, Earth-Science Reviews, 126(321-357).
Wyatt, M., & Curry, J. (2014, May). Role for Eurasian Arctic shelf sea ice in a secularly varying hemispheric climate signal during the 20th century. Climate Dynamics, 42(9-10), 2763-2782. Retrieved from https://link.springer.com/article/10.1007/s00382-013-1950-2#page-1
Wyser, K., Noije, T. v., Yang, S., Hardenberg, J. v., & Declan O’Donnell, a. R. (2020). On the increased climate sensitivity in the EC-Earth model from CMIP5 to CMIP6. Geosci. Model Dev., 13, 3465-3474.
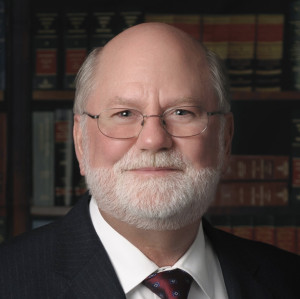
Andy May, now retired, was a petrophysicist for 42 years. He has worked on oil, gas and CO2 fields in the USA, Argentina, Brazil, Indonesia, Thailand, China, UK North Sea, Canada, Mexico, Venezuela and Russia. He specializes in fractured reservoirs, wireline and core image interpretation and capillary pressure analysis, besides conventional log analysis. He is proficient in Terrastation, Geolog and Powerlog software. His full resume can be found on linkedin or here: AndyMay